Introduction
In the nearly 70 years since John McCarthy coined the term “artificial intelligence,” we’ve seen wave after wave of technological innovation, from the rise of personal computing to the internet, mobile devices, the cloud, and now, generative AI.
Each of these shifts has created new opportunities and new questions for leaders. How can these technologies help my organization thrive? What new possibilities could they offer? How should I organize for optimal impact? And, perhaps most importantly, how can I use these technologies in a way that promotes trust among customers, citizens, patients, partners, shareholders, and the public?
In this article, we’ll share insights from organizations, analysts, AI leaders inside and outside of the company.
Finally, we’ll offer suggestions for pragmatic next steps to accelerate your progress, whether you’re just getting started, developing your organizational expertise, or well along the path.
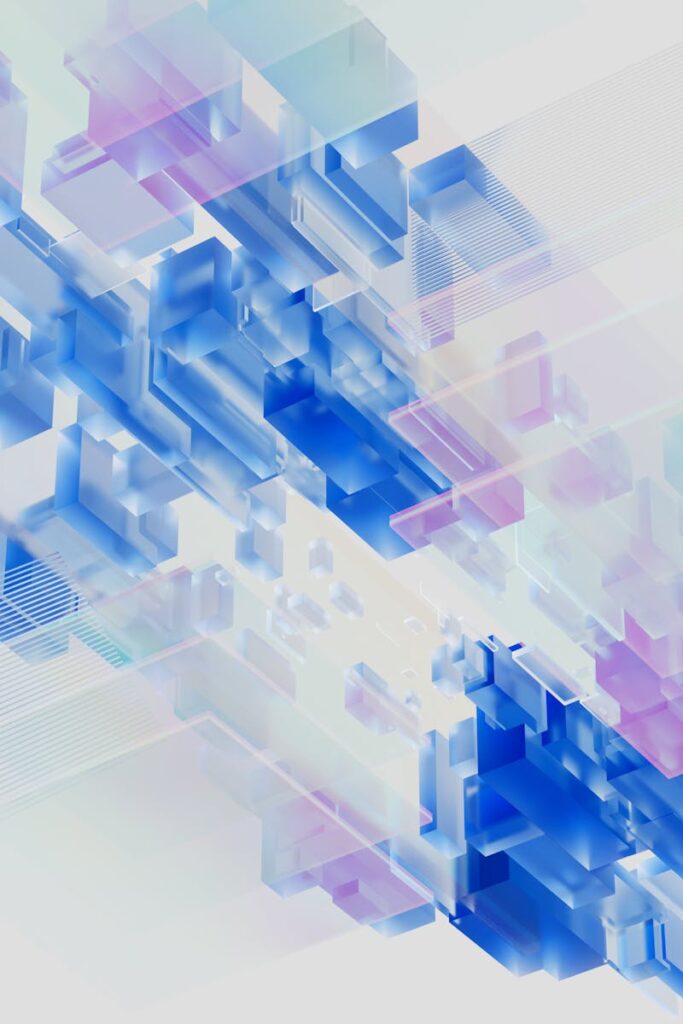
Opportunity of generative AI
While the term “artificial intelligence” has been around since the 1950s, the pace of AI innovation has accelerated in the past several years, thanks to plentiful data, greater access to computing power, and the size and sophistication of algorithmic models.
AI is fundamentally different from technologies that have come before it, both in its approach and its capabilities. It is based on probabilities, rather than rules, and “learns” from data without requiring explicit instructions. AI makes it possible for computers to perform certain tasks that previously only humans could do, such as visual perception, speech recognition, decision-making, and language translation.
Generative AI takes that one step further. It can be used for, among other things, content creation, summarization, and question answering, as well as the creation of images based on text prompts, all using everyday language. “For the first time, there will be a way for every human being to express their intent to a computing device in very natural ways,” says Kevin Scott, Executive Vice President and Chief Technology Officer at Microsoft.
Given the pace of AI innovation, the most frequent questions we hear from companies are: Where do I go from here? How do I create the most impact? What does success look like?
While there is no one answer for all organizations, we’re starting to see best practices emerge across five discrete categories, which we refer to here as the five pillars of AI success.
Stage 1: Exploring
Learning about AI and experimenting with it in some parts of the organization.
Stage 2: Planning
Actively assessing, defining, and planning AI strategy across the organization.
Stage 3: Formalizing
Formalizing, socializing, and executing on AI strategy across the organization and in multiple business units while starting to realize value.
Stage 4: Scaling
Delivering both incremental and new value across the organization.
Stage 5: Realizing
Realizing consistent AI value across the organization and in multiple business units.
Business strategy
Clearly defined and prioritized business objectives, use cases, and measurement of AI value
One of the biggest drivers of AI success is the degree to which the organization has defined and prioritized business objectives, use cases, and how it will measure value. This is particularly important given the wide applicability of AI to so many different needs, such as process optimization, content generation, summarization, procurement, supply-chain optimization, and more. Success requires rigorous focus on strategic goals as well as a growth mindset to embrace challenges and learn from failure.
“Rather than starting by asking what AI can do, we need to turn the telescope around and ask, ‘What are you trying to do in your business, and how can AI help?’” says Jason Price, Director of Specialist Management at Microsoft.
In fact, the Gartner® 2022 AI Use-Case ROI Survey states that the “main barriers preventing implementation of AI are unable/hard to measure value and lack of understanding AI benefits and uses.”
Nineteen percent of respondents cited “unable/hard to measure the value,” and 19 percent cited “lack of understanding AI benefits and uses.”
In Quick Answer: What Is the True Return on AI Investment? Gartner stated: “Enterprises do not achieve maximum leverage from artificial intelligence investments, despite increased spending. Executive leaders must become keen and discerning creators of
AI investment strategies in order to obtain optimum value from AI initiatives,” and that “the best return yield from AI investment will come from an extensive portfolio of AI, guided by an expansive and evolving investment thesis that is aligned to strategic priorities and helps to allocate resources based on business impact. Organizations that follow a portfolio management plan to determine most AI use cases are 2.4 times more likely to reach ‘mature’ levels of AI implementation.”
Technology strategy
An AI-ready application and data platform architecture, aligned parameters for build vs. buy decisions, and plans for where to host data and applications to optimize outcomes
The pace of AI innovation has captured the imaginations of people around the world. It has also intensified many of the biggest questions leaders face when seeking to optimize AI value, such as:
- Do I have the infrastructure required for AI applications to access data securely, quickly, and at scale?
- Based on my top-priority use cases, should I buy, build, or modernize AI applications?
- How should I determine whether to host data and AI applications on-premises or in the cloud?
As you work to resolve these questions, the first step is to choose an application and data platform architecture that will meet your organization’s requirements. Your architecture will determine the technologies you need, whether you buy a prebuilt solution, build it yourself, or opt for a combination.
“You can’t democratize AI if you don’t have an architecture that connects everyone across the company,” says Andy Markus, Chief Data Officer at AT&T. “The cloud makes that possible.”
AI strategy and experience
A systematic, customer-centric approach to AI that includes applying the right model to the right use case and experience in building, testing, and realizing AI value across multiple business units, use cases, and dimensions
Customer-centricity and taking a systematic approach to AI are both emerging as key contributors to AI success. The 2023 Gartner® report Survey Analysis: AI-First Strategy Leads to Increasing Returns found that “41 percent of mature AI organizations use customer success-related business metrics,” while “the strategic importance of AI techniques has once again been confirmed by our respondents. 77 percent of mature organizations adopt an AI-first strategy, systematically considering AI for every use case.”
Another critical driver of AI success is applying the right model to your use case— in other words, using the right tool for the right job—to solve specific problems and realize value. This applies whether you’re buying applications or building or your own.
Additional factors that tend to correlate with an organization’s AI success include:
- The number of AI use cases deployed.
- The length of time they’ve been in use.
- The degree to which they have scaled across the business.
- The degree of value they have generated, measured by productivity, revenue, or another metric.
These factors can also reveal potential barriers to success. One of the most common examples is the “perpetual proof of concept” loop, which tends to point to gaps in alignment between projects and valued business outcomes. Finally, the degree to which AI is democratized throughout the organization – extending AI capabilities and tools from a small group of experts to the organization as a whole – is also a leading indicator of success.
Organization and culture
A clear operating model, leadership support, change-management process, access to continuous learning and development, and strong relationships with diverse subject-matter experts.
In conversations with customers and partners, organization and culture frequently emerge as critical factors for success.
Operating model
According to Gartner: “The pace of AI technology maturation and diverse approaches make it difficult to capture and sustain value from AI initiatives. Effective AI operating models that leverage current investments in people, processes, and technologies enable IT leaders to drive successful AI initiatives.” It can mean the difference between AI projects that are viewed as science experiments and those that become significant value-drivers.
Salim Naim, Director of Specialist Management at Microsoft, says one of the key questions to consider “is whether your operating model is geared towards just experimentation and centralized, or whether it is designed to be embedded in every aspect of the business.” He advises organizations to ensure they’re taking an inclusive approach to developing their operating model. Leaders should ask themselves: “What should my operating model be that allows business units and different geographies in global organizations to adopt it?”
Leadership support
In discussions with customers and partners and in our own experience of AI transformation, we have found that organizations that derive the most value from AI are typically those whose leadership recognizes and supports the opportunities of AI with words, resources, and actions.
This may start as an initiative from the top or as a grassroots effort that gradually gains momentum. Either way, says Andreas Nauerz, Chief Technical Officer at Bosch Digital, “You need an atmosphere which encourages people to experiment and learn, even from failure. This is especially crucial considering the pace of technological advancement.”
More about how Microsoft customers are approaching AI transformation in their organization and culture, read “Lessons from Enterprise AI Pioneers” in the Wall Street Journal.
Change management
An organization’s ability to manage change is also a critical driver of AI success. “You very quickly learn that by the time you succeed with something, it’s already outdated,” says Mikkel Bernt Buchvardt, Director of Data and Analytics at SEGES Innovation. He suggests embracing this reality rather than letting it slow you down. “You can keep gold-plating your methods, or you can make it good enough to deliver some value.”
Skill-building and learning
Access to skill development, continuous learning, and certifications are also key. But according to Salim Naim, AI success isn’t simply skill acquisition – it needs to become a more sustainable capability within your organization. “As you mature, you go beyond what you solve to how well you solve it,” he says.
Strong relationships with subject-matter experts
Access to technologists with the right skills in the right roles is also fundamental to success. But it’s just as important to foster relationships with subject-matter experts across a spectrum of competencies to ensure that AI projects truly serve business objectives. As Andy Markus says: “The very first step of the journey is not even technical. It’s to establish a great partnership with the business. The number one goal is to deliver value to the company and to our customers. Sure, we’re technologists, and we can get really jazzed about doing cutting-edge things with technology. But the ultimate reason we’re here is to deliver value for our company and to our customers.”
AI governance
Implementation of processes, controls, and accountability structures to govern data privacy, security, and responsible use of AI
“Don’t ask what computers can do. Ask what they should do.” Microsoft President Brad Smith wrote those words about the ethics of AI in a book he co-authored in 2019. “This may be one of the defining questions of our generation,” the authors declared.
Four years later, AI – and its relationship to trust, data privacy, and security – is on the minds of people and organizations around the world. “Consumer faith in cybersecurity, data privacy, and responsible AI hinges on what companies do today,” a recent McKinsey report stated.
As with any consequential new technology, AI must be built on a foundation of security, risk management, and trust. “Ensuring the right guardrails for the responsible use of AI will not be limited to technology companies and governments,” wrote Antony Cook, Corporate Vice President and Deputy General Counsel at Microsoft, in a recent blog post announcing Microsoft’s AI customer commitments. “Every organization that creates or uses AI systems will need to develop and implement its own governance systems,” he said.
Organizations seeking to reap the greatest benefit from AI must develop their understanding of the data governance, security, and responsible AI implications of their decisions, with regard to both risks and opportunities.
Since 2017, Microsoft has been sharing expertise, providing training curriculum, and creating dedicated resources to support responsible use. “A theme that is core to our responsible AI program and its evolution over time is the need to remain humble and learn constantly,” says Natasha Crampton, Chief Responsible AI Officer at Microsoft. “Responsible AI is a journey, and it’s one that the entire company is on.”
For insight on Microsoft’s approach to Responsible AI, read “The building blocks of Microsoft’s responsible AI program.”
Getting started
Use this guide to help identify priority areas as you define your AI strategy and roadmap
Suggested next steps | |
Business strategy | • Define and prioritize business objectives such as customer experience, productivity, revenue growth, employee experience, and other key goals. • Determine how you will measure the value of those objectives. • Identify and prioritize AI use cases that support your goals. • Build a portfolio management plan to help guide your investments. |
Technology strategy | • Based on your top-priority use cases, determine whether to buy, modernize, or build applications. • Assess whether you have the infrastructure for AI applications to access data securely, quickly, and at scale. • Consider the scalability and performance implications of hosting data and AI applications on-premises or in the cloud. • Ensure your cloud infrastructure is built to run large AI workloads and deliver reliability at scale. • Evaluate your organization’s Zero Trust security posture. • Explore how to use AI for improving security, in terms of deploying and protecting organizational assets, developing and maintaining policies and procedures, and monitoring and responding to incidents or emerging threats. |
AI strategy and experience | • Familiarize yourself with generative AI use cases and how they might support your business needs. and • Develop a systematic process to consider AI for every use case. • Assess the number of business units and processes, length of time in production, and age of deployments in your organization to reveal patterns that may point to opportunities or blockers. • Build intelligent apps on your data to improve the intelligence and relevance of model outputs. • Consider using Microsoft 365 Copilot, or build your own copilot to accelerate learning and time to value. |
Organization and culture | • Define your operating model for AI. • Secure—or develop a plan to secure—leadership support backed by resources. • Develop strong relationships with a diverse range of subject-matter experts in the business. • Strengthen your organization’s ability to manage change. • Identify and implement the right learning and skill-building paths in place. • Approach AI as a sustainable capability within your organization and culture. |
AI governance | • Review and share resources on responsible use of AI to identify the models and approaches that best suit your organization. • Consider the enablement model that best fits your needs, such as hub-and-spoke, centralized, or distributed. • Consider the principles of secure AI and how to ensure your data is protected end to end from platform to applications and users. • Consider the processes, controls, and accountability mechanisms that may be required to govern the use of AI, and how AI may affect data privacy and security policies. |
Summary
Given the speed of AI innovation and the uniqueness of every organization, no single framework could possibly account for all organizations, regions, or circumstances. But we hope that these pages have helped you identify potential areas of strength and opportunities to accelerate your AI innovation journey.